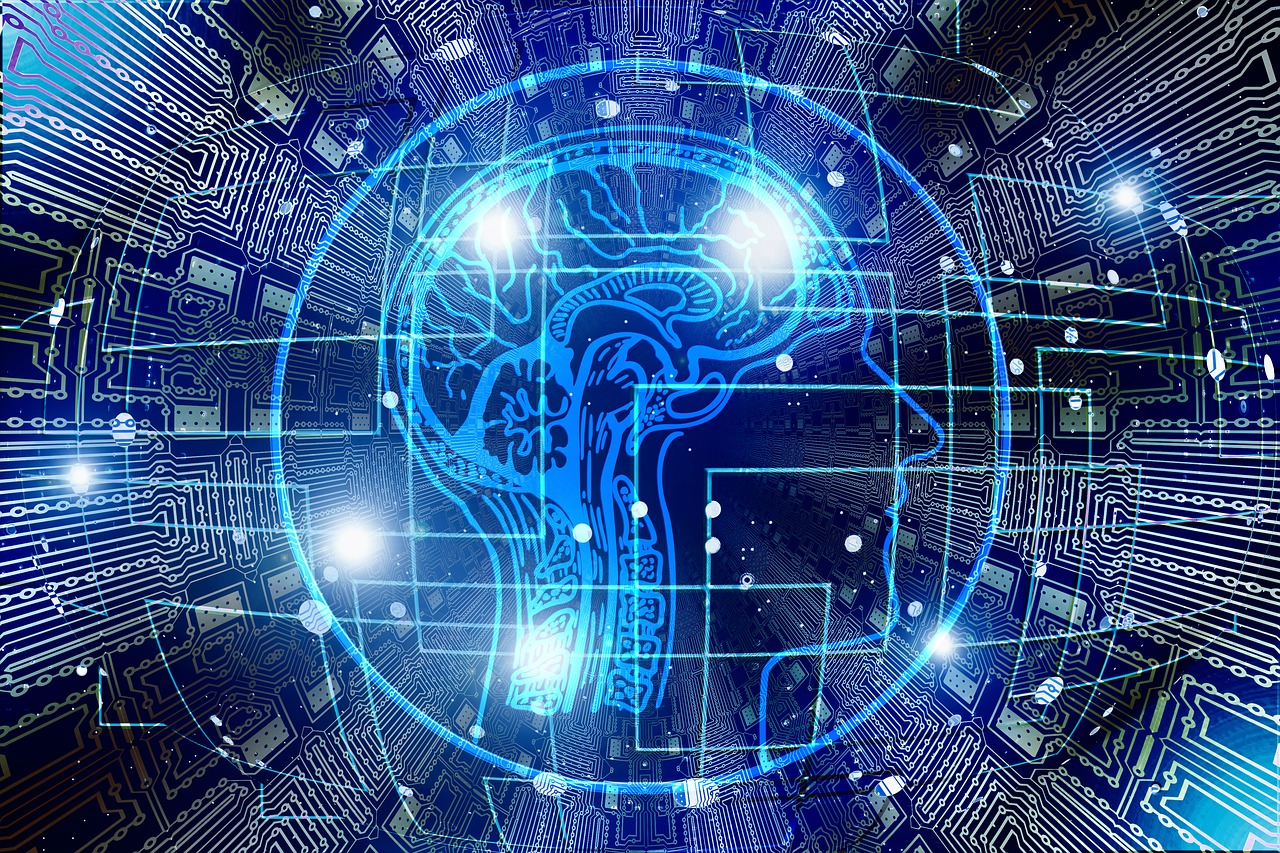
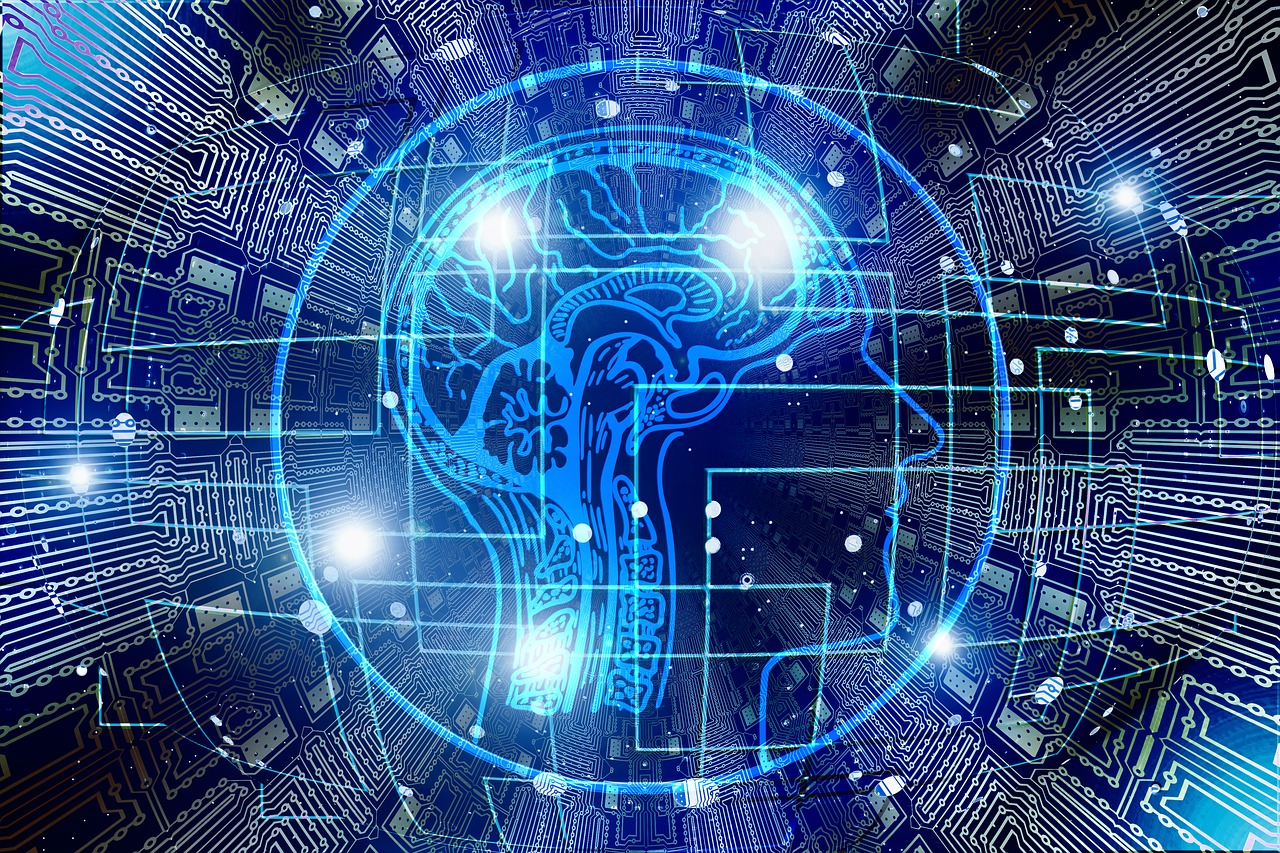
Artificial intelligence is all the rage. Today, computing can perform feats that appear similar to stuff humans might do, if we were smarter –that is, if we had more computing power. But just because a computing system can do something, should it? What actions do we want computers performing on our behalf?
We can treat this like a question of “agency.” Not unlike a nonemployee insurance agent that provides customer services on behalf of an insurance company, we are venturing into an area in which computing systems will be expected to perform work – to act – on behalf of brands, or other legal entities.
Artificial intelligence has demonstrated that it can perform incredible processing feats, such as recommending music, recognizing faces and predicting part failures, by recognizing patterns in data. Moreover, it can do these things at scale, with a proficiency that’s at least equal to a group of humans, and at very low cost.
But most of these tasks involve trivial agency questions. As AI is put into service to perform more complex tasks, where tradeoff options are unresolvable or even can’t be formulated, agency becomes a central feature of system design, construction and operation. Indeed, artificial intelligence success is spawning artificial agency problems.
The algorithms for determining if a task is or is not simple, and therefore if agency is or isn’t a potential problem, are really weak. Generally, the rule has been that if agency questions are a problem, don’t implement AI. That’s why so many of today’s powerful AI solutions are operating in domains that already accept automation from engineering, legal, and moral perspectives, but are looking for better approaches to automate.
But we know that’s going to change. Elon Musk’s decision to add self-driving capability to Tesla vehicles didn’t involve a lot of public discussion, to the best of my knowledge. His “algorithm” appears to have been “I shoot first and ask questions later.” Interestingly, Musk is one of the loudest voices in the pitched debate about limiting AI, essentially claiming that AI will bury humanity one way or another. Perhaps Musk figured that by moving first, he could both reap extraordinary profits and get a jump on ingratiating himself to his future robot masters.
More likely Musk, like many others seeking greater public discourse about complex topics, wants a bit of government cover as he seeks to push AI’s limits. But in any event more discussion is necessary – especially at smaller scale, like inside companies. Here are some of the questions that business leaders have to start addressing:
AI is automating increasingly complex work. However, business leaders need to look closely at AI agency questions. Just because an AI system can be built doesn’t mean it should be deployed.
THANK YOU